In 1950, Alan Turing suggested The Imitation Game, a test of whether a machine can exhibit intelligence indistinguishable from that of a human. Five years later, the term artificial intelligence (AI) was first used by John McCarthy in the description of a field dedicated to the creation of intelligent machines. Today, AI is a rapidly evolving field with many subsets, the majority of which apply machine learning (ML), a technique by which a computer can learn to perform a task without explicitly being taught how to. The numerous applications of machine learning include fields such as natural language processing, speech recognition and modern computer vision. These are already part of our daily lives: in your smartphone you will find examples in the way of predictive text, virtual assistants and face recognition. Many scientific disciplines have benefited from the rise of deep learning (DL), a subset of machine learning that focuses on the use of large artificial neural networks, allowing for processing of data on greater scales and at more complex levels [Figure 1].1 2
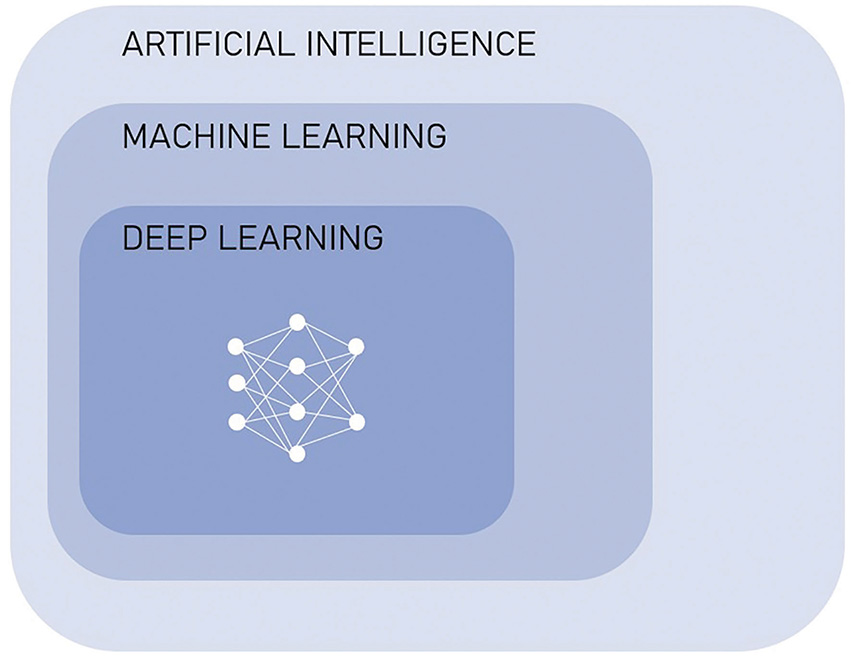
Figure 1. Relationship between artificial intelligence, machine learning and deep learning
Healthcare providers now appreciate that this technology can be utilised in clinical medicine to enhance the way we diagnose, make decisions and treatment plans, as well as monitor and predict outcomes. There has been an exponential increase in research related to medicine and AI over the past decade, with a significant contribution by radiology and ophthalmology.3 4 Image interpretation relies greatly on pattern recognition and this, in combination with the availability of large databases of images, creates an ideal environment in which to apply deep learning in the field of computer vision.5 A 2021 Australian survey highlighted that 15.7% of ophthalmologists and 6.1% of radiologists reported the use of AI in their daily clinical practice.6
Similar to image interpretation, CTG analysis is heavily based on pattern recognition and with high inter-clinician variability, is a task where AI could standardise and assist in decision making. Machine learning for CTG interpretation has been examined as early as 1989, with many of the more recent algorithms demonstrating high accuracy comparable to clinician interpretation.7 However, when applied, a significant difference in neonatal outcomes is yet to be demonstrated and it is likely to be some time before it is available in daily clinical practice.8 9 It is important to note that, aside from the potential to enhance knowledge and improve outcomes, AI has a role to play in increasing efficiency and automating tasks.
In a global setting, where cervical cancer is a major public health problem, AI may be applied in diagnostics to assist the process of cervical screening, especially where access to laboratory resources is limited. Deep learning analysis of digitalised cytology could be impactful in this situation, and there have been promising results in testing of algorithm accuracy, as well as practical application in a low-income setting.10 11 Further to this, automated visual evaluation is also being examined in the analysis of cervical photography to detect pre-cancerous change.12 Ongoing development of this could allow for efficient point-of-care testing with simple equipment and rapid availability of results.
Machine learning can also be used to make predictions, which may assist in individualised risk assessment. An Australian group created a deep learning algorithm which was able to predict up to 45% of stillbirths in the test population, based on background characteristics and antenatal complications.13 Another study included fetal biometry and umbilical artery doppler measurements in addition to demographics, which enabled prediction of 75% of stillbirths secondary to impaired placentation.14 There are many models utilising deep learning for stillbirth prediction, which will require ongoing collaborative research and consideration as to how they might influence antenatal monitoring and intervention.
The above examples highlight that there are, of course, many barriers to the practical application of AI in medicine, including economic and ethical implications. One main reason why medicine may be hesitant to accept this technology in clinical practice is the lack of explainability, or the ‘black box’ nature of deep learning models.15 These algorithms are often extraordinarily complex, to the extent that the process of obtaining a result or outcome cannot be interpreted, and the model cannot explain itself. Without this understanding, to what extent can we comprehend and apply this information, or explain it? ‘Dr Roboto says so’ is not an ideal answer and highlights why this technology can be considered more of an adjunct than a replacement.
This links into another major concern, which is the potential for bias. Most machine learning models in medicine utilise supervised learning, meaning that the system uses training data that has been labelled by humans, and is therefore both limited and subject to human influence [Figure 2].16 17 Further, machine learning systems in numerous fields have been found to exhibit sex, race, social and other biases, which often stem from underrepresentation or misrepresentation in the underlying data.18 An example is of Amazon’s machine learning hiring system, which, largely due to existing male dominance in the technology industry, was actively penalising female and preferentially selecting male applicants.19 It is known that sex-based bias is an ongoing issue in medical research but with regards to AI, a model presented with an imbalanced dataset can make skewed decisions that become lost in the ‘black box’, which are especially difficult to identify and can result in potentially unrecognised underperformance in relation to that group. Bias needs to be carefully anticipated and addressed in AI research so that in application, it does not further perpetuate or widen existing disparities.
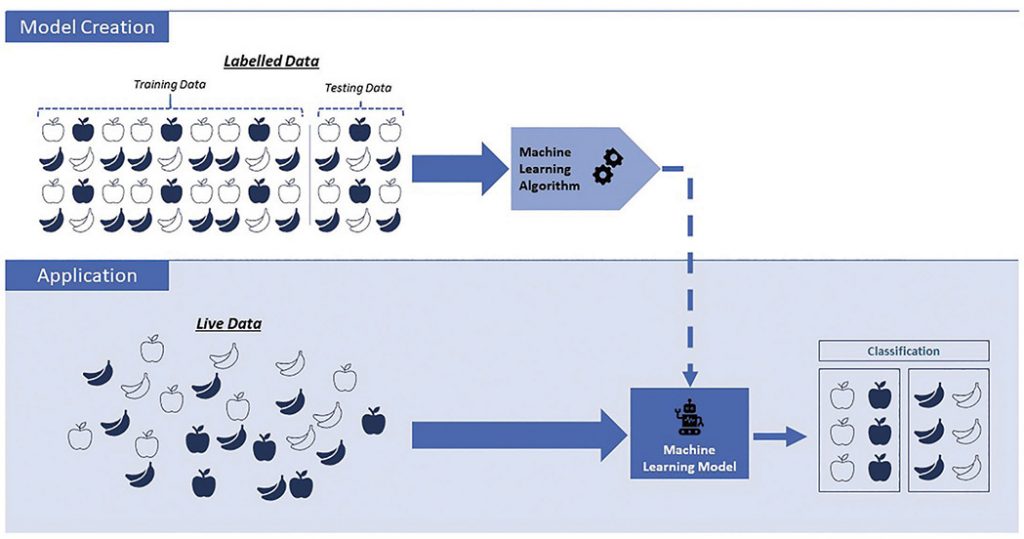
Figure 2. Representation of supervised Machine Learning
There are a great number of potential applications of AI to O&G – further examples of current research areas include algorithms for prediction of preterm labour, gestational diabetes and IVF outcomes, ovarian cancer screening and prognostication, visual evaluation to aid embryo selection, and many more.20 Machine learning algorithms will continue to improve with more time and data, and, when it comes time for incorporation into practice, it will ultimately be up to clinicians to ensure safety and applicability. It will be our responsibility to understand the principles which will enable us to assess, enhance and then one day welcome this technology.
Special thank you to Danny for verifying the technicalities, and to Marilla for her enthusiasm!
References
- Drukker L, Noble JA, Papageorghiou AT. Introduction to artificial intelligence in ultrasound imaging in obstetrics and gynecology. Ultrasound Obstet Gynecol. 2020;56:498-505.
- Garcia-Canadilla P, Sanchez-Martinez S, Crispi F, et al. Machine Learning in Fetal Cardiology: What to Expect. Fetal Diagn Ther. 2020;47:363-372.
- Drukker L, Noble JA, Papageorghiou AT. Introduction to artificial intelligence in ultrasound imaging in obstetrics and gynecology. Ultrasound Obstet Gynecol. 2020;56:498-505.
- Scheetz J, Rothschild P, McGuinness M, et al. A survey of clinicians on the use of artificial intelligence in ophthalmology, dermatology, radiology and radiation oncology. Sci Rep. 2021;11(1):5193.
- Drukker L, Noble JA, Papageorghiou AT. Introduction to artificial intelligence in ultrasound imaging in obstetrics and gynecology. Ultrasound Obstet Gynecol. 2020;56:498-505.
- Scheetz J, Rothschild P, McGuinness M, et al. A survey of clinicians on the use of artificial intelligence in ophthalmology, dermatology, radiology and radiation oncology. Sci Rep. 2021;11(1):5193.
- Garcia-Canadilla P, Sanchez-Martinez S, Crispi F, et al. Machine Learning in Fetal Cardiology: What to Expect. Fetal Diagn Ther. 2020;47:363-372.
- Garcia-Canadilla P, Sanchez-Martinez S, Crispi F, et al. Machine Learning in Fetal Cardiology: What to Expect. Fetal Diagn Ther. 2020;47:363-372.
- Balayla J, Shrem G. Use of artificial intelligence (AI) in the interpretation of intrapartum fetal heart rate (FHR) tracings: a systematic review and meta analysis. Arch Gynecol Obstet. 2019;300(1):7-14
- Bao H, Sun X, Zhang Y, et al. The artificial intelligence-assisted cytology diagnostic system in large-scale cervical cancer screening: A population-based cohort study of 0.7 million women. Cancer Med. 2020;9(18):6896-6906.
- Holmström O, Linder N, Kaingu H. Point-of-Care Digital Cytology With Artificial Intelligence for Cervical Cancer Screening in a Resource-Limited Setting. JAMA Netw Open. 2021;4(3):e211740.
- Hu L, Bell D, Antani S, et al. An Observational Study of Deep Learning and Automated Evaluation of Cervical Images for Cancer Screening. J Natl Cancer Inst. 2019;111(9):923-932.
- Malacova E, Tippaya S, Bailey HD, et al. Stillbirth risk prediction using machine learning for a large cohort of births from Western Australia, 1980–2015. Sci Rep. 2020:10(1);5354.
- Akolekar R, Tokunaka M, Ortega N, et al. Prediction of stillbirth from maternal factors, fetal biometry and uterine artery Doppler at 19-24 weeks. Ultrasound Obstet Gynecol. 2016:48(5);624-630.
- Drukker L, Noble JA, Papageorghiou AT. Introduction to artificial intelligence in ultrasound imaging in obstetrics and gynecology. Ultrasound Obstet Gynecol. 2020;56:498-505.
- Drukker L, Noble JA, Papageorghiou AT. Introduction to artificial intelligence in ultrasound imaging in obstetrics and gynecology. Ultrasound Obstet Gynecol. 2020;56:498-505.
- Garcia-Canadilla P, Sanchez-Martinez S, Crispi F, et al. Machine Learning in Fetal Cardiology: What to Expect. Fetal Diagn Ther. 2020;47:363-372.
- Cirillo D, Catuara-Solarz S, Morey C, et al. Sex and gender differences and biases in artificial intelligence for biomedicine and healthcare. NPJ Digit Med. 2020;3:81.
- Goodman, R. Why Amazon’s Automated Hiring Tool Discriminated Against Women. American Civil Liberties Union. October 12, 2018. Available from: www.aclu.org/blog/womens-rights/womens-rights-workplace/why-amazons-automated-hiring-tool-discriminated-against
- Iftikhar P, Kuijpers MV, Khayyat A, et al. Artificial Intelligence: A New Paradigm in Obstetrics and Gynecology Research and Clinical Practice. Cureus. 2020;12(2):e7124.
Leave a Reply